27% Reduction of NSFs for Leading Small Dollar Lender
Discover how a top consumer lender leveraged Pave's Income Prediction Model to reduce NSFs by 27%, improving user satisfaction and repayment outcomes.
Goal
Reduce NSFs
Problem
The lender faced high NSF rates because loan payments were scheduled at times when borrowers did not have funds available.
Solution
By leveraging Pave’s Income Prediction Model, the lender was able to align loan payments with borrowers’ paychecks, reducing NSFs by 27%.
Challenge
Loans on auto-pay frequently lead to Non-Sufficient Funds (NSFs) when borrowers’ accounts lack the necessary funds on the designated payment day. This often stems not from a borrower’s overall inability to pay but from a timing mismatch between payment schedules and cashflow, causing complications and additional costs for both parties involved.
Our customer aimed to prevent NSFs by aligning payment schedules with when borrowers are most likely and able to pay—their payday.
Challenges Presented by Failed Payments:
- Direct Costs: Each failed payment incurs transaction and balance inquiry fees.
- Poor Customer Experience: Frustrates borrowers, damaging their relationship with the lender.
- Payment Hierarchy: Misaligned payments decrease the lender’s priority for repayment amongst other financial obligations.
- Increased Delinquencies: Correlation with the likelihood of overall borrower delinquency and/or default.
By proactively addressing the issue of failed payment attempts, lenders can significantly ease financial strain and operational challenges. This strategy plays a crucial role in diminishing the overall rates of delinquency. In turn, this fosters improved financial well-being and a more positive experience for both lenders and borrowers.
Approach
To address this, the lender employed Pave’s Income Prediction Model designed to align loan repayment with a borrower’s income schedule.
Our Income Prediction Model detects paychecks in bank transactions and predicts the exact day the user will get paid with over 90% accuracy, allowing lenders to increase the success of their collections and reduce NSFs.
By adopting the Income Prediction Model, the lender quickly overcame the challenge of building a complex predictive model from scratch, allowing them to align collections with borrowers’ income schedules in just weeks.
Result: Lender Achieved 27% Reduction in NSFs
The lender’s implementation of the model resulted in a 27% reduction in NSFs, enhancing operational efficiency and improving customer experience while promoting financial health.

Conclusion
Both risk teams and borrowers struggle with traditional payment schedules, which aren’t optimized for repayment. Pave’s Income Prediction Model simplifies aligning payments with borrowers’ likelihood and ability to pay. Teams can deploy Pave’s Cashflow Analytics and Scores in under two weeks, reducing losses, and helping borrowers to pay on time and improve their financial health.
For more information on Pave’s solution for payment plans, download our Collections Whitepaper, or contact us at howdy@pave.dev to book a demo.
Explore Use Cases
Cash Advance
Score users to increase approvals, advance amounts, and improve repayments.
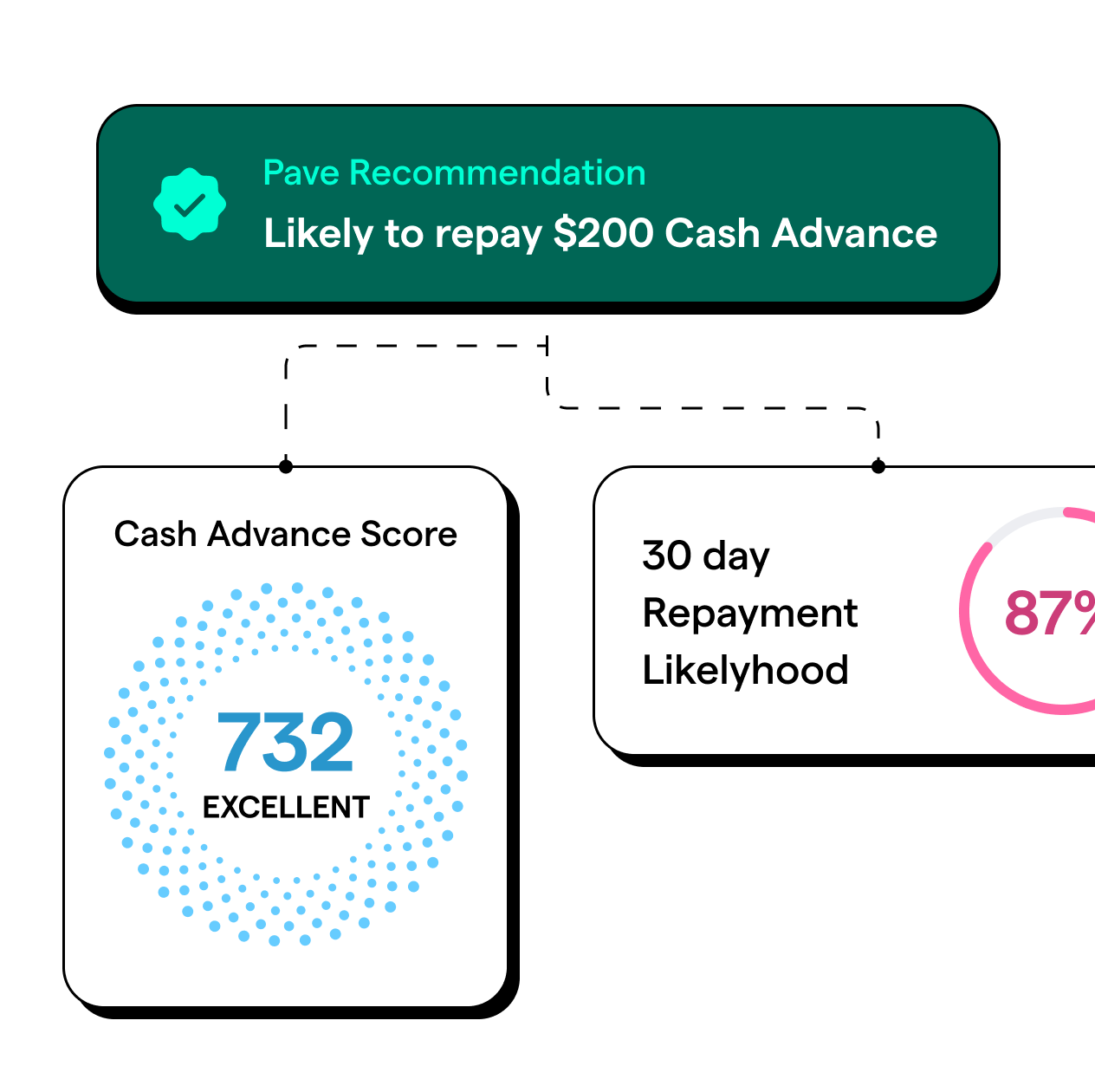
Personal Loans
Identify users with high likelihood of making the first 4 payments to reduce delinquencies.

Charge Cards
Graduate users to higher secured or unsecured limits based on increased affordability.

Credit Cards
Set dynamic credit limits based on users' income and affordability.
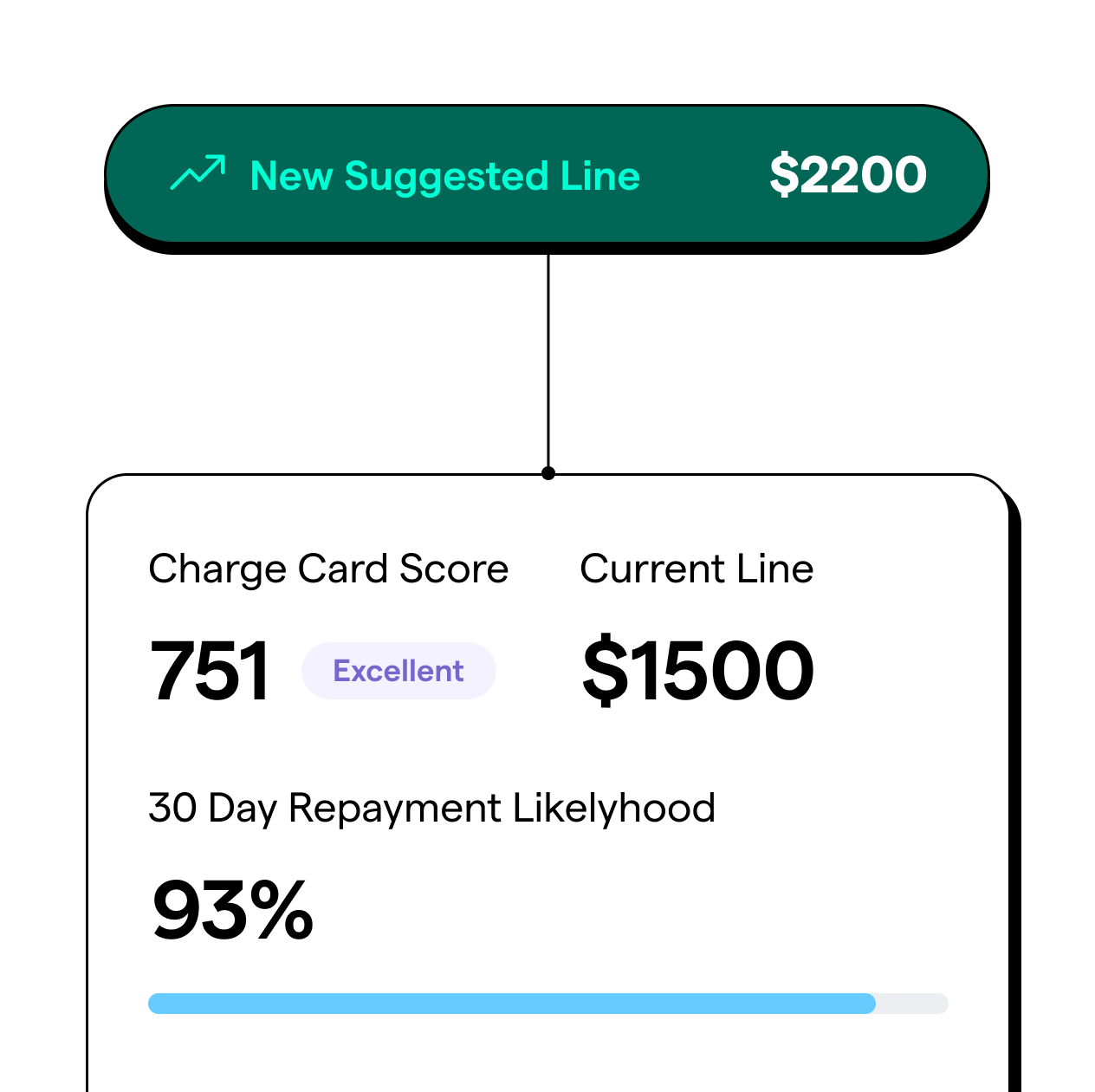
Drive growth with Cashflow-driven Analytics
Use our Cashflow-driven Attributes and Scores to provide timely, borrower-specific insights tailored to your lending criteria. Make informed decisions that enhance approval rates and loan performance.